Substantial amounts of subsurface data on the Norwegian Continental Shelf have been and are increasingly collected.
Blog post
The blogpost is written by
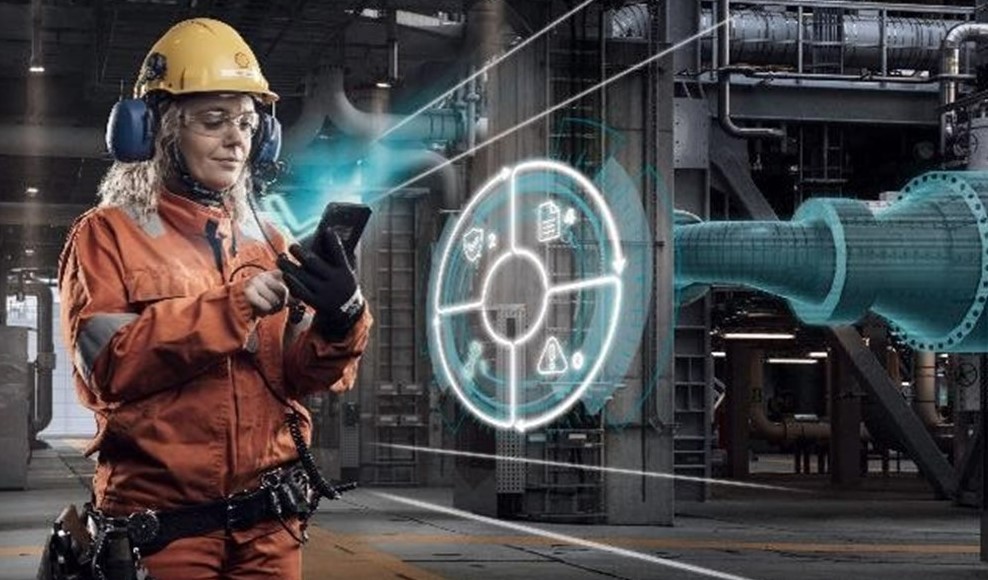
Data acquisition, sharing and fast access have been important contributions to the success of the petroleum industry in Norway. Complex and large models for multiple scenarios require high performance computing. Improved input to models using conventional methods or assisted with ML requires underlying data and training data sets based on easily accessible and exchangeable data from multiple data sources. The industry is evolving fast with new solutions in cloud computing technology development and standardization such as the OSDU community for subsurface data sharing standard, DELFI, etc..
Large scale applied data science will shift from computing and analysis at a single site to the new paradigm of federated computing among multiple sites that support transferring computation to data, rather than the opposite. This technological revolution offers a great opportunity to improve the current state-of-the-art tools in both better process modelling and computing performance. In addition, the new coming Cloud-Edge-IoT (Internet of Things) technology with automated federated data service for accessing data sources across multiple sites, sufficient computing power for handling of intensive computations for model development and testing with realistic field cases is offering a major step towards data utilization and effectivization (Zhao et al., 2010). Data assimilation (DA) is a robust reservoir management modelling framework for optimization, decision making and value creation of subsurface resources (Evensen, 2021; Chen and Oliver, 2017). DA has led to a paradigm shift for the way reservoir models have been used for reservoir characterization and production forecast, and many companies now use the ensemble workflows in their operations (Hanea et al., 2015).
The competing strategies to properly account for multiple scenarios (Höge et al., 2020) are computationally challenging. The anticipated sustainable subsurface value chain, by including other reservoir usages such as CO2 and H2 storage, energy efficiency related to injection and production, and handling fluctuation of renewable energy source introduces a new level of complexity. Consequently, new methods are needed for obtaining reliable models, like the rapidly growing interest in machine learning (ML) (Luo et al., 2021) and multi-fidelity (MF) modeling (Peherstorfer et al., 2018; Nezhadali et al., 2021). Furthermore, computational challenges associated with Bayesian Model Evidence (BME) also need to be handled (Sinsbeck et al., 2021). One route of research is the inclusion of physics-based, ML-based, and hybrid proxy models in Bayesian learning. The well-established ensemble DA and optimization methods can be extended to cover the complete sustainable value chain linking the subsurface, well, and injection lines and provide optimal decision methods.